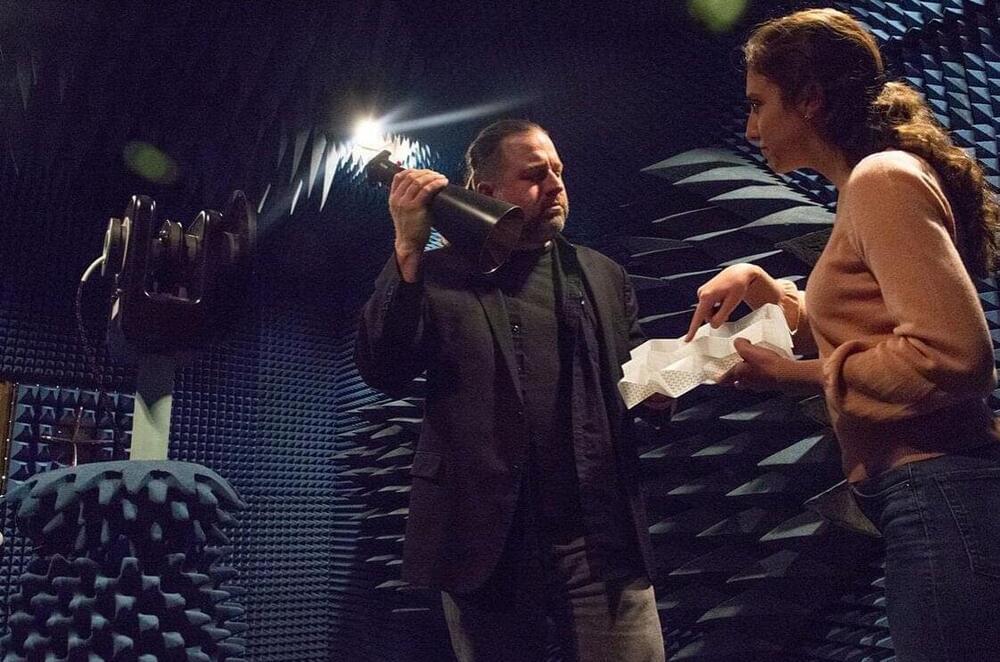
Additive manufacturing, or 3D printing, can create custom parts for electromagnetic devices on-demand and at a low cost. These devices are highly sensitive, and each component requires precise fabrication. Until recently, though, the only way to diagnose printing errors was to make, measure and test a device or to use in-line simulation, both of which are computationally expensive and inefficient.
To remedy this, a research team co-led by Penn State created a first-of-its-kind methodology for diagnosing printing errors with machine learning in real time. The researchers describe this framework—published in Additive Manufacturing —as a critical first step toward correcting 3D-printing errors in real time. According to the researchers, this could make printing for sensitive devices much more effective in terms of time, cost and computational bandwidth.
“A lot of things can go wrong during the additive manufacturing process for any component,” said Greg Huff, associate professor of electrical engineering at Penn State. “And in the world of electromagnetics, where dimensions are based on wavelengths rather than regular units of measure, any small defect can really contribute to large-scale system failures or degraded operations. If 3D printing a household item is like tuning a tuba—which can be done with broad adjustments—3D-printing devices functioning in the electromagnetic domain is like tuning a violin: Small adjustments really matter.”